3 Principles for Successful AI Integration in Your Product Strategy
- Nishant Saxena
- Mar 6, 2024
- 3 min read
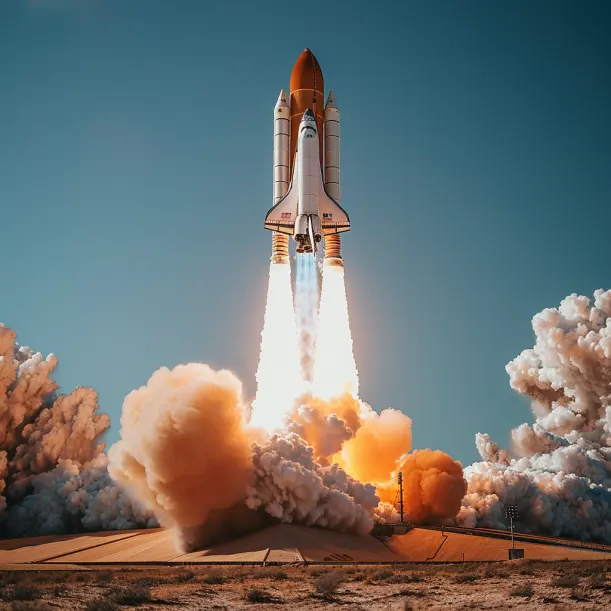
Technology often moves at a faster pace than businesses can keep up with, but AI is a whole new beast. It is already playing a huge role in shaping consumer behavior and business trends, and the rate of advancement is staggering. It represents both unprecedented opportunities and formidable challenges: A recent [survey by Workday]( https://newsroom.workday.com/2023-06-28-Workday-Global-Survey-Majority-of-Business-Leaders-Believe-Humans-Should-be-Involved-in-AI-Decision-Making-Cite-Ethical-and-Data-Concerns ) found that 73% of business leaders feel pressure to implement AI in their organization, but 72% say their organization lacks the skills needed to do so. This predicament intensifies when we consider product strategy, as AI accelerates the speed of delivering products while simultaneously amplifying uncertainty around which solutions will triumph.
The challenge for businesses isn't just adopting AI technology, it's weaving AI into the fabric of their products in a way that enhances user experience, drives innovation, and creates competitive advantage. This involves not only understanding the various forms and applications of AI, but also recognizing its potential to revolutionize development, customization, and engagement.
So, how can businesses navigate the challenges of this rapid technological evolution and capitalize on the opportunities, and potential market value, presented by it? My experience leading numerous AI initiatives as a product leader and business development consultant has taught me that keeping pace with AI is not just a matter of implementing it. It’s about identifying how the technology can benefit users and add value, deploying it strategically, and embracing a culture of continuous improvement. Here we’ll explore what many leaders are doing wrong and I’ll share three core principles to align AI integration with product strategy.
AI Definitions and Applications
For business leaders, the key is to not think about AI as a piece of technology, but instead as a strategic asset that, when used responsibly and effectively, can lead to significant advancements in operations, customer experience, and decision making. To leverage AI successfully, leaders first need to understand its forms and applications. Here are some definitions:
Artificial Intelligence (AI): At its core, AI aims to mimic human intelligence. This includes tasks such as learning, reasoning, problem solving, and understanding language.
General vs. Narrow AI:
Artificial General Intelligence (AGI): Still only hypothetical, AGI would be capable of performing any intellectual task that a human can do, covering a broad range of expertise across multiple domains. Companies like Google and OpenAI are heavily investing in exploring AGI.
Narrow AI: Narrow AI excels in performing a specific task, such as spam detection, facial recognition, or data analysis. It’s important to note that AI proficient in one task may not necessarily excel in another.
Machine Learning (ML): A significant subset of AI, ML enables machines to learn from data without being explicitly programmed. It focuses on using algorithms to parse data, identify patterns, and make decisions. In essence, it's about teaching machines to learn from experience. Netflix, for example, uses a browsing system that analyzes data such as a customer's viewing history and the preferences of similar viewers in order to create personalized recommendations.
Deep Learning (DL): A subset of ML, deep learning uses neural networks, inspired by the human brain, to simulate human-like thinking. This approach allows machines to process large data sets and is pivotal in applications such as image recognition and voice assistants. For example, Google Photos employs deep learning to categorize images, allowing users to search for specific objects, scenes, or faces. This is achieved by training neural networks on millions of pictures, enabling the differentiation of elements like cats from dogs or landmarks like the Eiffel Tower.
Large Language Models (LLM): LLMs, like ChatGPT, are foundation models that process extensive text data. They are commonly utilized in customer service, content creation, and even software development.
Current common use cases for AI in business include automating repetitive tasks, increasing efficiency, creating content, and generating insights from vast data sets. Marketing, sales, product, business development, operations, hiring - virtually every department can be improved or positively disrupted.
For product teams specifically, AI can provide insights drawn from user data, enabling them to tailor experiences and anticipate customer needs with unprecedented precision. From Netflix recommendations to Google Photos intuitive image categorization, AI is redefining the parameters of functionality and interaction.
Beyond its impact on consumer-facing products, AI is also revolutionizing B2B and internal products. Companies are leveraging AI to create intelligent supply chain systems that can predict disruptions, optimize inventory, and streamline logistics. AI algorithms can identify patterns and anomalies that would be impossible for humans to detect, enabling businesses to make proactive, data-driven decisions. This not only enhances operational efficiency but also contributes to a more resilient and responsive supply chain.
At every stage of the product lifecycle—from ideation and development to launch and continuous improvement—AI stands as a potent catalyst for innovation. Its integration, however, must be guided by a clear vision, strategic alignment with business goals, and a relentless focus on delivering value to the end user.
留言